Blogs
Services
Introduction to Machine Learning
BY QDegreesPUBLISHED
May. 02, 2023
May. 02, 2023
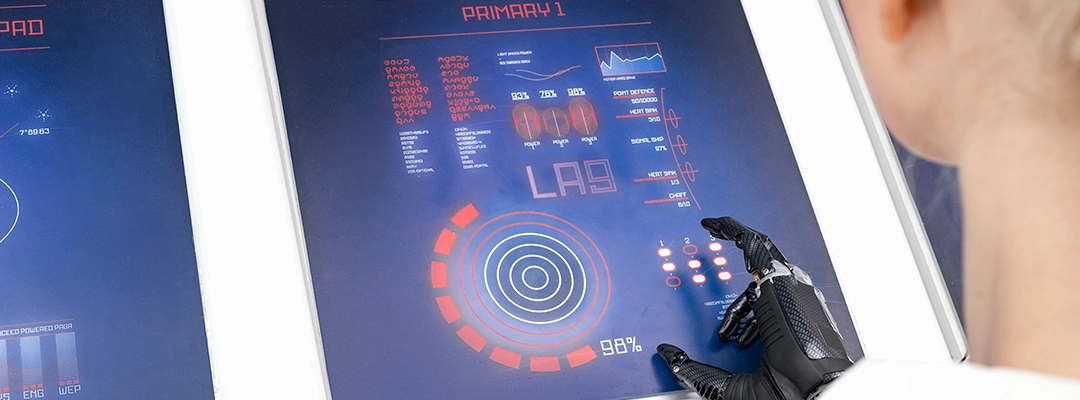
Machine learning has grown dramatically in popularity in recent years, propelled by developments in analytics and programming, as well as improved datasets and the development of artificial brains.
Whether you recognize it or not, machine learning is now present in various applications today, including automated interpreting, picture identification, voice search, self-driving automobiles, and more.
Let's get started with knowing the basics of Machine Learning, why it's used, how it works, and the relevant concepts.
What is Machine Learning? Why Is It Important?
With the use of Machine Learning (ML), a form of Artificial Intelligence (AI), computer programs may anticipate outcomes more accurately without having to be explicitly instructed to do so. In order to forecast new output values, machine learning algorithms use previous information as inputs. Machine learning is frequently used in engines that make recommendations. Business process automation (BPA), maintenance planning, spam screening, ransomware prevention, and identifying fraudulent activity are a few other common uses.
Deep learning is fundamentally a neural framework with three layers and is a subset of machine learning. These neural connections make an effort to mimic how the human brain functions; however, they fall well short of replicating it, enabling it to "learn" from vast quantities of data.
Machine learning is essential because it aids in creating new goods and provides businesses with a picture of trends in consumer behavior and operational and economic patterns. A significant portion of the activities of many of today's top businesses, like Uber, Facebook, and Google, revolve around the use of machine learning. For many businesses, machine learning has emerged as a key differentiation factor in competition.
Types of Machine Learning
The way in which a prediction-making algorithm works to improve its accuracy is a common way to classify traditional machine learning. There are four fundamental strategies: supervised learning, unsupervised learning, semi-supervised learning, and reinforcement learning. The kind of data that data scientists wish to forecast determines the kind of algorithm they use.
1. Supervised ML
For such types of machine learning, data analysts describe the parameters that they want the computer to look for correlations between and provide its models with classified training data. The algorithm's input and output are both described.
2. Un-supervised ML
Algorithms used in this kind of machine learning are trained on unlabeled data. The program searches through sets of data in search of any significant relationships. The input data that algorithms use to train and the predictions or suggestions they provide as output are predefined.
3. Semi-supervised ML
The above-stated two forms of machine learning are integrated with this type of ML. A computer program can be fed chiefly annotated training data by data analysts. Still, the program has the ability to examine the data by itself and come to its own conclusions about the data set.
4. Reinforcement ML
Data analysts frequently use reinforcement machine learning to train a system to finish a multi-step operation with well-defined criteria. Data analysts frame an algorithm to fulfill an objective, and they provide it with positive or negative feedback as it demonstrates how to do so. However, the algorithm often chooses the course of action on its own.
Importance of Humans in Machine Learning
Whenever an ML model is advanced, it might be challenging to explain how it operates. Because the company must be able to justify every action, data analysts in specific vertical sectors are forced to employ basic machine learning algorithms. Complex models can generate precise forecasts, but it can be challenging to describe how the output was calculated to a layperson.
Future of Machine Learning
Although machine learning programs have been available for a while, they have recently gained widespread acceptance. Artificial intelligence has become more well-known and offers incredible future potential for any area, most notably business. Deep learning models primarily power modern AI applications. With AI becoming increasingly accessible, reaching common users through top players, and bridging the gap of technology, it is surely going to be game-changing for both brands and consumers. The faster businesses innovate their products with AI integration, the better their chances of staying ahead in the race to retain customers in this fast-paced world.